SeMOpt
We are excited to release Atinary’s new ML algorithm and research paper on Semantic Memory Enhanced Optimization (SeMOpt, link to paper). SeMOpt is Atinary’s transfer-learning algorithm to accelerate optimization processes by leveraging existing knowledge learned from past experiments and existing databases. It automatically identifies key information and guides the optimization algorithms to the most promising parameter […]
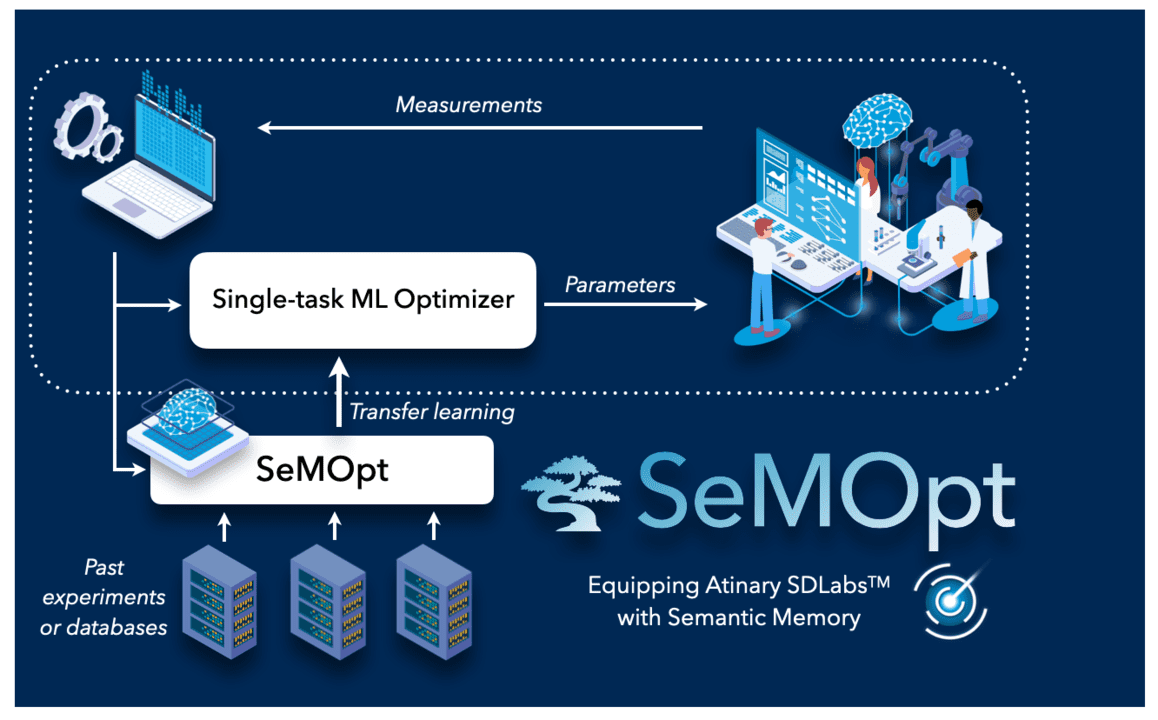
We are excited to release Atinary’s new ML algorithm and research paper on Semantic Memory Enhanced Optimization (SeMOpt, link to paper). SeMOpt is Atinary’s transfer-learning algorithm to accelerate optimization processes by leveraging existing knowledge learned from past experiments and existing databases. It automatically identifies key information and guides the optimization algorithms to the most promising parameter region from the start. SeMOpt works hand-in-hand with any general-purpose Bayesian optimization ML algorithms.
SeMOpt strengthens Atinary offering of data-driven ML solutions to further accelerate R&D of new molecules and materials. SeMOpt overcomes limitations of ML optimization algorithms by using state-of-the-art techniques from meta-learning and few-shot learning. These techniques implicitly learn inductive biases that strongly resemble concepts in experimental science. This learned intuition is then used to strengthen and guide optimization processes on novel tasks.
We expect SeMOpt to provide opportunities to accelerate R&D across the experimental sciences. Sample applications of SeMOpt accelerated include the following optimization challenges:
- parameters for accurate dispensing of liquids and solids by robotic platforms;
- chemical reactions with novel (unstudied) catalysts and/or ligands and/or substrates with access to yield measurements from related reactions;
- design of drug delivery systems for novel pharmaceutical compounds.
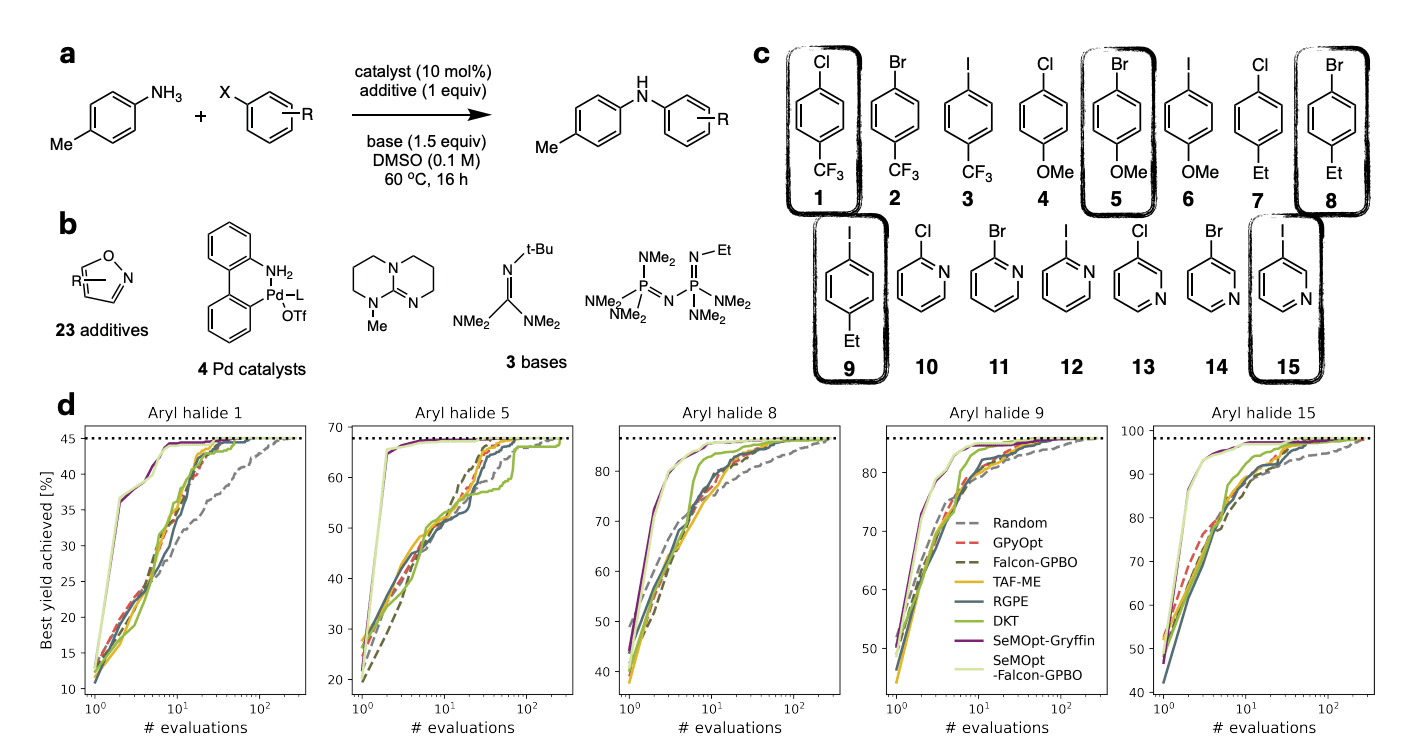
The paper presents SeMOpt’s performance on the optimization of five simulated cross-coupling reactions, as well as on the palladium-catalyzed Buchwald-Hartwig cross-coupling of aryl halides with 4-methylaniline. These chemical reactions are widely used in organic synthesis, with application across industrial sectors, including pharma and biotech.
SeMOpt accelerates the optimization rate by a factor of 10 or more compared to standard single-task ML optimizers (those without transfer learning capabilities). Moreover, the case studies presented show that SeMOpt outperforms several existing ML Bayesian optimization strategies that leverage historical data.
Our new algorithm is a valuable technical contribution for general-purpose optimization and provides further support of the benefits from replacing the traditional trial-and-error experimentation process with Atinary Self-Driving Labs® technology, now powered with semantic memory.
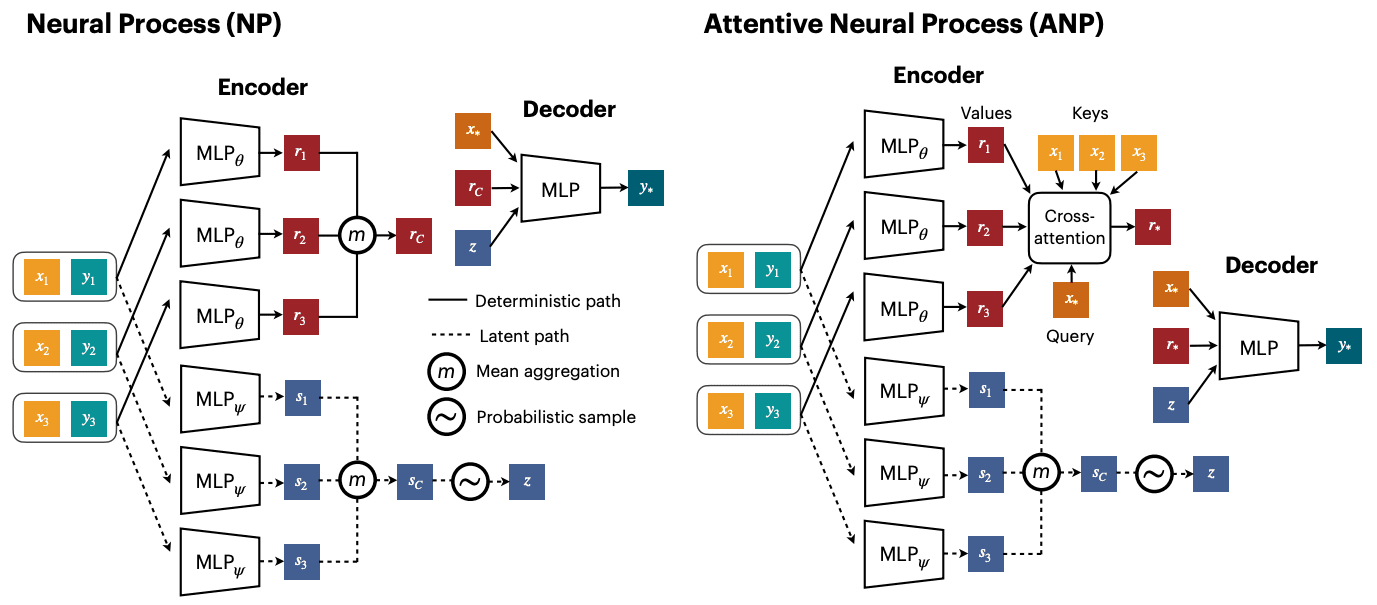
Read more about it here: https://chemrxiv.org/engage/chemrxiv/article-details/6276f20987d01f0f03dcbe10