Leveraging HTE to optimize yield in deprotection reaction with Takeda Pharmaceuticals
Integrating Atinary's SDLabs platform for AI-driven HTE to maximize yields from under 50% to above 90% after 3 iterations.
Overview
Troc (2,2,2-Trichloroethoxycarbonyl) deprotection is a critical step in organic synthesis, particularly in peptide and nucleoside chemistry, where it is used to remove protective groups from amines and hydroxyl groups. The process typically involves the use of a reducing agent (i.e. zinc) to remove the Troc group, releasing the free amine or hydroxyl functionality.
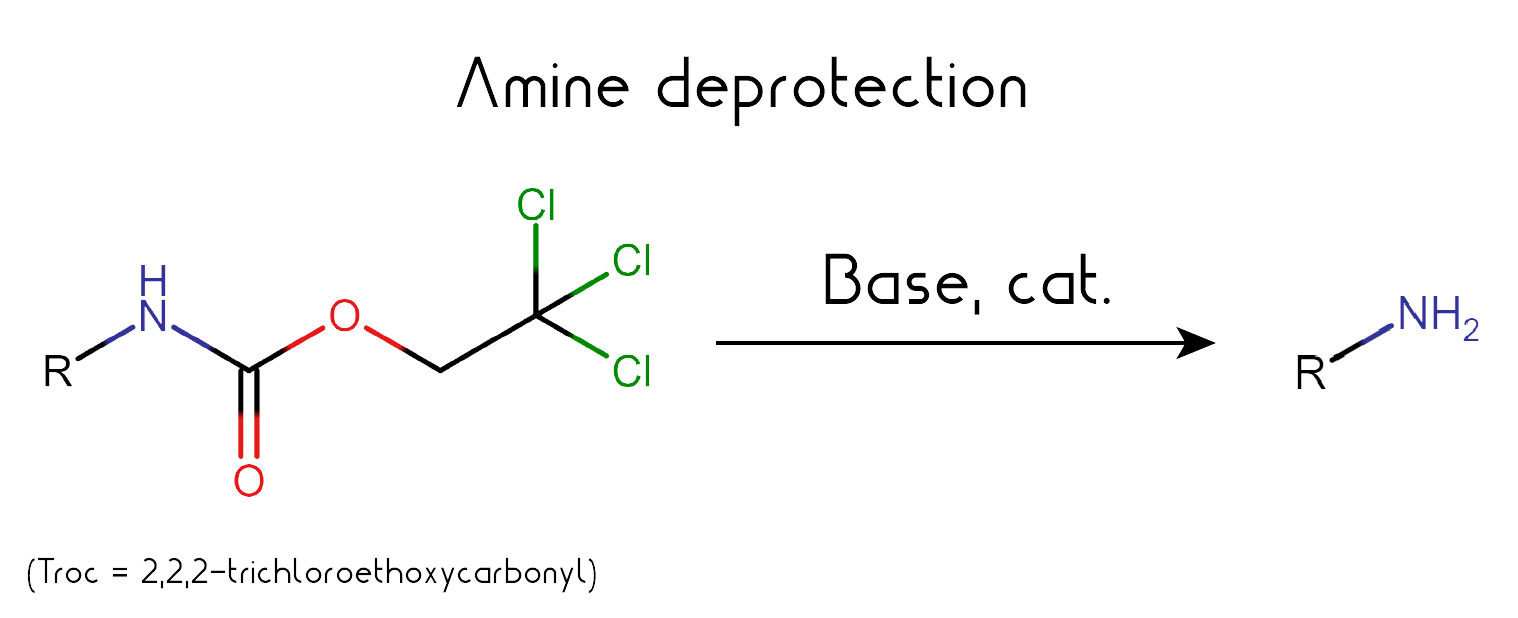
Challenges
Reaction kinetics, chemoselectivity and by-product formation are few reasons why this reaction typically has a low yield. The efficiency of Troc deprotection depends on several variables, including the choice of solvent, reaction temperature, and the concentration and type of catalyst and reagents used. Optimizing these variables can be cumbersome but is essential to achieving high yields and selectivity in Troc deprotection.
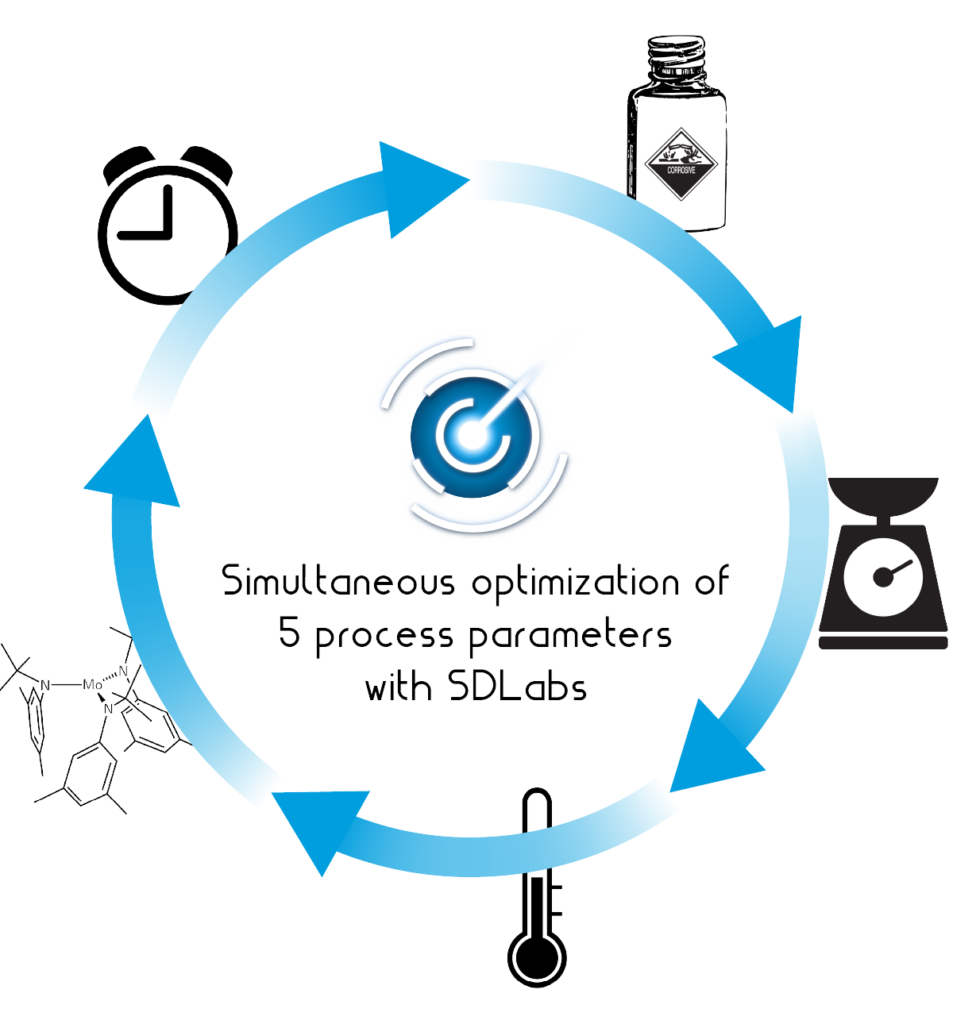
Schematic diagram illustrating the five process variables (the type and amount of base, temperature, catalyst, and reaction time) as inputs into Atinary’s SDLabs platform for AI-driven optimization of Troc deprotection.
Use Case
In this specific case of Troc deprotection, the initial reaction efficiency was approximately 50%, which was deemed insufficient for the desired outcome. To improve this, we executed an AI-driven optimization process, focusing on five critical variables that were identified to have the most significant impact on the reaction’s success: the type and amount of base, temperature, catalyst, and reaction time. In total, this represented about 600 parameter combinations.
Benefits
Traditional approaches to reaction optimization, such as trial-and-error or Design of Experiments (DoE), are time-consuming, costly, and resource-intensive, potentially requiring hundreds of experiments to identify optimal conditions. Whereas using AI-technology, after conducting only two generations or iterations of 16 experiments each, orchestrated in Atinary’s no-code AI platform, SDLabs, our algorithm was able to suggest several experimental sets of parameters leading to a yield above 90%.
Graphical representation of the use of Atinary’s SDLabs platform in minimizing experimentation to quickly reach maximum yield after two iterations. The traffic light colour code indicates the progress towards reaching the objective of maximum yield.
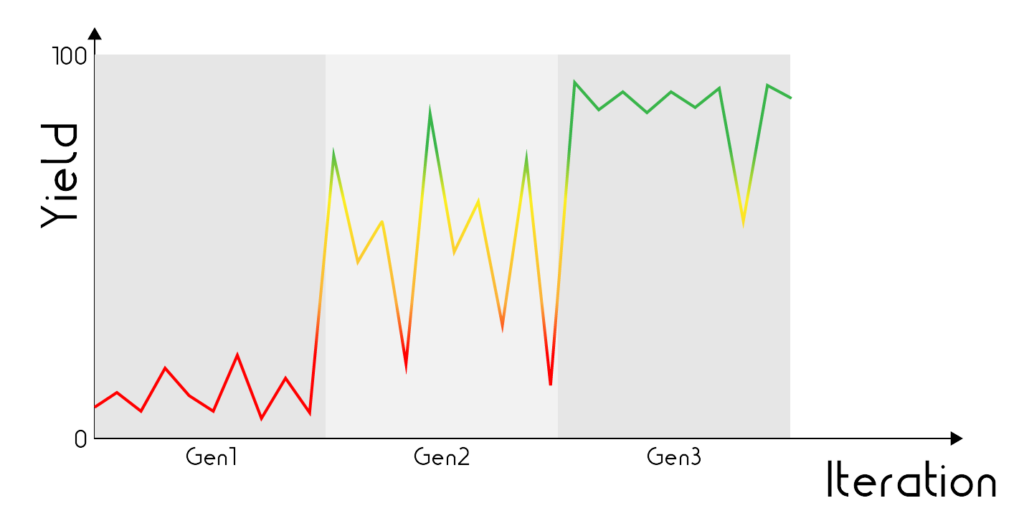
Resources
- Link to Press Release
- Link to C&EN Webinar: Leveraging HTE & Automation for Synthetic Molecule Process Development (sponsored by Unchained Labs)
- Link to ACD/Labs-Atinary Webinar: DMTA Innovation at Takeda – Digitalized, AI-Augmented, Autonomous (hosted by ACD/Labs)
- More about Takeda Pharmaceuticals
- Download use case as a pdf