Smart Chemical Library Screening and Ligand Optimization
Leveraging AI to maximize yield, while minimizing experiments and cost.
Overview
One common use-case in chemical synthesis is the screening of a large chemical library to identify compounds that achieve a specific goal, such as maximizing reaction yield or optimizing selectivity. This process may involve evaluating hundreds of potential candidates with diverse chemical structures and properties to find the best performing compounds.
Challenge
One of the primary challenges in screening a large chemical library is managing the vast size of the library as it would be too costly and time consuming to test all the elements. Moreover, each chemical possesses many descriptors, such as molecular weight, polarizability, and electronic properties. Thus, it is a complex challenge to leverage all descriptors effectively and find significant correlations that meet the specific goal. Additionally, the vast amount of data generated can be overwhelming, making it challenging to identify trends and correlations without advanced analytical tools.
Use Case
The goal is to screen a large chemical library and find the ligands with the maximum conversion and optimal selectivity. Each ligand has a unique set of chemical descriptors. In addition, some compounds used in the original product formulations were no longer available to buy in the market. To solve this additional challenge, researchers used SDLabs to identify replacement chemicals that lead to the same results and performance.
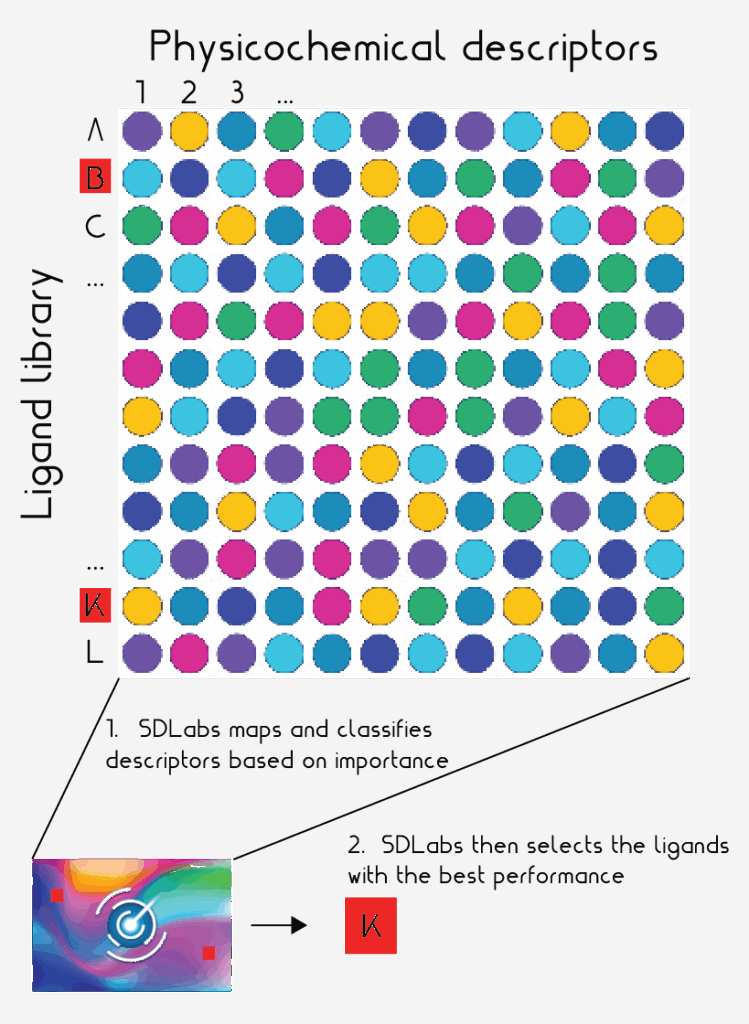
A schematic diagram of a ligand library and AI-driven screening by Atinary’s SDLabs. Each ligand (represented along each row as A, B, C, …) possesses a unique set of chemical descriptors (depicted along each column 1, 2, 3, …) that becomes a fingerprint for each ligand. Atinary’s machine learning (ML) algorithms within the SDLabs platform can process and analyze vast amounts of data, from each ligand’s fingerprint. First, SDLabs will map and classify descriptors based on the importance of the objective. Then, SDLabs will select the best performing ligand (red box). As an example, Ligand B and Ligand K are predicted by SDLabs as promising ligands.
Benefits
Using SDLabs to execute the AI-driven optimization of catalysts from a large chemical library offers significant advantages. Atinary’s machine learning (ML) algorithms can process and analyze vast amounts of data, identifying complex, non-linear relationships between chemical descriptors and catalytic performance that might be overlooked by traditional methods, such as Design of Experiments (DoE). In less than two months, by executing Bayesian Optimization strategies that learn from past experiments, Atinary’s ML model was able to identify the most promising ligands that maximized the yield, while minimizing the number of experiments and the cost.
Resources
- Download the use case as a pdf